Invited Speakers
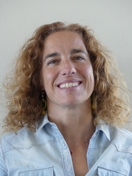
Professor, Department of Information Systems, Decision Sciences and Statistics, ESSEC Business School, France
Co-authors:
Ola Jabali, Department of Electronics, Information and Bioengineering, Politecnico di Milano, Italy
Andrea Mor, Department of Economics and Management, University of Brescia, Italy
Alberto Simonetto, Multiprotexion srl, Gropello Cairoli, Italy
M.Grazia Speranza, Department of Economics and Management, University of Brescia, Italy
Talk Title
The Bi-objective Long-haul Transportation Problem on a Road Network
Abstract
Long-haul truck transportation is concerned with freight transportation from shipments’ origins to destinations, with vehicle trips lasting from some hours to several days. Drivers performing long-haul transportation are subject to strict rules derived from Hours of Service (HoS) regulations. There exists a large body of literature integrating HoS regulations within long-haul transportation. The optimization problems in this context generally deal with routing and scheduling decisions aimed at determining where a driver should stop and how long a rest should be. However, the overwhelming majority of the literature on long-haul transportation ignores refueling decisions and treats fuel costs as proportional to the traveled distance. In this talk we analyze a long-haul truck scheduling problem where a path has to be determined for a vehicle traveling from a specified origin to a specified destination. We consider refueling decisions along the path while accounting for heterogeneous fuel prices in a road network. Furthermore, the path has to comply with Hours of Service (HOS) regulations. Therefore, a path is defined by the actual road trajectory traveled by the vehicle, as well as the locations where the vehicle stops due to refueling, compliance with HOS regulations, or a combination of the two. This setting is cast in a bi-objective optimization problem, considering the minimization of fuel cost and the minimization of path duration. An algorithm is proposed to solve the problem on a road network. The algorithm builds a set of non-dominated paths with respect to the two objectives. Given the enormous theoretical size of the road network, the algorithm follows an interactive path construction mechanism. Specifically, the algorithm dynamically interacts with a geographic information system to identify the relevant potential paths and stop locations. Computational tests are made on real-sized instances where the distance covered ranges from 500 to 1500 km. The algorithm is compared with solutions obtained from a policy mimicking the current practice of a logistics company. The results show that the non-dominated solutions produced by the algorithm significantly dominate the ones generated by the current practice, in terms of fuel costs, while achieving similar path durations. The average number of non-dominated paths is 2.7, which allows decision-makers to ultimately visually inspect the proposed alternatives.
Assistant Professor, EECS department at Syracuse University, USA
Talk Title
Constrained-based Differential Privacy
Abstract
Data sets and statistics about groups of individuals are increasingly collected and released, feeding many optimizations and learning algorithms. In many cases, the released data contain sensitive information whose privacy is strictly regulated. Differential privacy has emerged as the de-facto standard to protect data privacy. Despite its large theoretical value, when private data sets are used as inputs to complex machine learning or optimization tasks, they may produce results that are fundamentally different from those obtained on the original data and even rise unintended bias and fairness concerns. In this talk, I will focus on the challenge of releasing privacy-preserving data sets for complex data analysis tasks. I will introduce the notion of Constrained-based Differential Privacy (C-DP), which allows casting the data release problem to an optimization problem whose goal is to preserve the salient features of the original data. I will review several applications of C-DP in the context of hierarchical census data, data streams, and energy systems. Finally, I will discuss the impact that differential privacy may have on the fairness of a downstream decision process and conclude with some open questions.
Jacobs Technion-Cornell Institute, Cornell Tech and Technion – IIT, New York, USA CERC, Polytechnique Montréal, Canada
Co-authors: Defeng Liu, CERC, Polytechnique Montréal, Canada
Talk Title
Learning in Local Branching
Abstract
Although state-of-the-art solvers for Mixed-Integer Programming (MIP) experienced a dramatic performance improvement over the past decades, the resolution of some MIPs is still challenging, requiring hours of computations while, in practice, high-quality solutions are often required to be computed within a very restricted time frame. In such cases, it might be preferable to provide anytime solutions, i.e., a first reasonable solution should be generated as early as possible, then better ones produced in the subsequent computation with the user deciding where to stop. In this respect, the local branching (LB) heuristic [2] was proposed to improve an incumbent solution either at very early stages of the computation within a general MIP framework or as a stand-alone algorithmic framework. Roughly speaking, given a feasible solution, the method iterates by first defining a solution neighborhood through the so-called local branching cut, then by exploring it by calling a black-box MIP solver. In the local branching algorithm, the choice of the neighborhood size is crucial to performance. In principle, it is desirable to have neighborhoods to be relatively small for efficient computation but still large enough to contain improving solutions. In [2], the size of the neighborhood is mostly initialized by a fixed constant value, then adjusted at run time. Nonetheless, it is reasonable to believe that there is no a priori single best neighborhood size and the choice of the value should depend on the characteristics of the problem. Furthermore, it is worth noting that, in many applications, instances of the same problem are solved repeatedly. Real-world problems have a rich structure: while more and more data points are collected, patterns and regularities appear. Therefore, problem-specific and task-specific knowledge can be learned from data and applied to adapting the corresponding optimization scenario. This motives a broader paradigm of sizing the solution neighborhoods in local branching. Following the line of work analyzed and surveyed in [1] on the use of Machine Learning (ML) for combinatorial optimization, in this work, we aim to guide the (local) search of the local branching heuristic by ML techniques. In particular, given a problem instance and a time limit for (heuristically) solving it, we exploit ML tools to predict reasonable good values of the neighborhood size, in order to maximize the performance of the local branching algorithm. We computationally show that the neighborhood size can indeed be learnt leading to improved performances and that the overall algorithm generalizes well both with respect to the instance size and, more surprisingly, across instances.
1 Yoshua Bengio, Andrea Lodi, and Antoine Prouvost. Machine learning for combinatorial optimization: a methodological tour d’horizon. European Journal of Operational Research, 290(2):405–421, 2021.
2 Matteo Fischetti and Andrea Lodi. Local branching. Mathematical programming, 98(1-3):23–47, 2003.